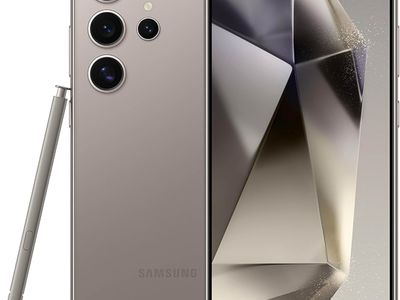
The Future of Jobs with AI: Unveiling the Endless Possibilities & Changes by ZDNet Experts

The Future of Jobs with AI: Unveiling the Endless Possibilities & Changes by ZDNet Experts
MR.Cole_Photographer/Getty Images
Building and rolling out AI-based systems might seem like a big and unwieldy project, fraught with risks. However, another mode of delivery is emerging: AI-based agents.
Generative AI has added new heft and capabilities to agents that have often been difficult to set up and manage. New research shows these easier-to-set-up services are capturing the attention of technologists and their business leaders.
Also: 6 ways AI can help launch your next business venture
AI-based agents represent the “next frontier” of AI, according to a report from consultant McKinsey. The report predicts the influence of agentic systems – defined as “digital systems that can independently interact in a dynamic world” – will increase.
Newsletters
ZDNET Tech Today
ZDNET’s Tech Today newsletter is a daily briefing of the newest, most talked about stories, five days a week.
Subscribe
While agentic systems have been around for some time, “the natural-language capabilities of gen AI unveil new possibilities, enabling systems that can plan their actions, use online tools to complete those tasks, collaborate with other agents and people, and learn to improve their performance,” the McKinsey team of authors, led by Lareina Yee, stated.
Also: This year’s top 8 use cases for AI
The next stage of generative AI is likely to be even “more transformative”, Yee and her colleagues suggested. “We are beginning an evolution from knowledge-based, gen-AI-powered tools – say, chatbots that answer questions and generate content – to gen AI-enabled agents that use foundation models to execute complex, multistep workflows across a digital world. In short, the technology is moving from thought to action.”
A majority of 1,100 tech executives (82%) responding to a recent survey from consultant Capgemini indicated they intend to integrate AI-based agents across their organizations within the next three years – up from 10% with functioning agents at the current time.
The report found seven in ten respondents (70%) would trust an AI agent to analyze and synthesize data, and 50% would trust an AI agent to send a professional email on their behalf. About three-quarters of respondents (75%) said they intend to deploy AI agents to tackle tasks such as generating and iteratively improving code. Other potential tasks for agents included generating and editing draft reports (70%) and website content (68%), and email generation, coding, and data analysis.
AI-powered agents are capable of taking on a diversity of roles. “A virtual assistant, for example, could plan and book a complex personalized travel itinerary, handling logistics across multiple travel platforms,” the McKinsey report said. “Using everyday language, an engineer could describe a new software feature to a programmer agent, which would then code, test, iterate, and deploy the tool it helped create.”
In another example, a vendor, Qventus , offers a customer-facing AI-based assistant called the Patient Concierge, which calls patients and reminds them of appointments, reiterates pre- and post-op guidelines, and answers general care questions.
Also: When’s the right time to invest in AI? 4 ways to help you decide
There are six levels of AI agents, each offering increasing functionality, as spelled out in this tutorial published by Amazon Web Services:
- Simple reflex agents: Suitable for simple tasks such as resetting passwords. “Operates strictly based on predefined rules and its immediate data. It will not respond to situations beyond a given event condition action rule.”
- Model-based reflex agents: Similar to simple reflex agents, but “rather than merely following a specific rule, evaluates probable outcomes and consequences before deciding. Builds an internal model of the world it perceives and uses that to support its decisions.”
- Goal-based/rule-based agents: Has more robust reasoning capabilities than the reflex agents, making them suitable for “more complex tasks such as natural language processing and robotics applications.” The goal/rules-based agent “compares different approaches to help it achieve the desired outcome, and always chooses the most efficient path.”
- Utility-based agents: “Compares different scenarios and their respective utility values or benefits” – such as helping customers search for the best airline deals. “Uses a complex reasoning algorithm to help users maximize desired outcomes.”
- Learning agents: “Continuously learns from previous experiences to improve its results. Using sensory input and feedback mechanisms, the agent adapts its learning element over time to meet specific standards. On top of that, it uses a problem generator to design new tasks to train itself from collected data and past results.”
- Hierarchical agents: This puts agents in charge of other agents. “The higher-level agents deconstruct complex tasks into smaller ones and assign them to lower-level agents. Each agent runs independently and submits a progress report to its supervising agent. The higher-level agent collects the results and coordinates subordinate agents to ensure they collectively achieve goals.”
Until now, software agents “have been difficult to implement, requiring laborious, rule-based programming or highly specific training of machine-learning models,” the McKinsey team noted. However, a major shift is now occurring.
“Gen AI changes that. When agentic systems are built using foundation models – which have been trained on extremely large and varied unstructured data sets – rather than predefined rules, they have the potential to adapt to different scenarios in the same way that LLMs can respond intelligibly to prompts on which they have not been explicitly trained.”
Also: 4 ways to help your organization overcome AI inertia
AI agents’ use of natural-language processing also changes the equation. “Currently, to automate a use case, it first must be broken down into a series of rules and steps that can be codified,” the McKinsey team said.
“These steps are typically translated into computer code and integrated into software systems – an often costly and laborious process that requires significant technical expertise. Because agentic systems use natural language as a form of instruction, even complex workflows can be encoded more quickly and easily. What’s more, the process can potentially be done by nontechnical employees, rather than software engineers.”
Artificial Intelligence
Photoshop vs. Midjourney vs. DALL-E 3: Only one AI image generator passed my 5 tests
AI-powered ‘narrative attacks’ a growing threat: 3 defense strategies for business leaders
Copilot Pro vs. ChatGPT Plus: Which AI chatbot is worth your $20 a month?
How my 4 favorite AI tools help me get more done at work
- Photoshop vs. Midjourney vs. DALL-E 3: Only one AI image generator passed my 5 tests
- AI-powered ‘narrative attacks’ a growing threat: 3 defense strategies for business leaders
- Copilot Pro vs. ChatGPT Plus: Which AI chatbot is worth your $20 a month?
- How my 4 favorite AI tools help me get more done at work
Also read:
- [New] Facebook's Videography Preference Which Orientation, In 2024
- [Updated] 2024 Approved Capture Your Gameplay Essential Webcams For Twitch Enthusiasts
- 2024 Approved Elevate Online Media Selecting the Best 5 Quality Boosters
- 2024 Approved Memetic Mastery Crafting Joy via Adobe
- Discover the Most Effective Cross-Platform Chat Applications for iOS and Android Devices
- Discover the Ultimate List of 8 Leading Projector Apps for Effortless Wi-Fi Hookup on Your Android Device
- Effortless Tricks for Bypassing Android Restrictions in Just a Few Taps
- Fine-Tuning Frames A Comprehensible Approach to iPhone Editing for 2024
- New Translate Live Videos With The Best Video Translator for 2024
- Overcoming Delays: A Comprehensive Guide to Getting Your Hands on Football Manager 2022
- Rescue Your Precious Moments: A List of the Best 6 iPhone Photography Recovery Tools
- Sluggish Mobile Data Woes: Quick Fix with These 10 Easy Steps
- The Ultimate List of Great Android Camera Apps for Amazing Shots
- The Ultimate List: 9+ Secure Unlocking Apps with Fingerprint Recognition for Android Smartphones
- Ultimate Review of the Shotcut App: Unveil Its Features and Benefits Now
- Title: The Future of Jobs with AI: Unveiling the Endless Possibilities & Changes by ZDNet Experts
- Author: Matthew
- Created at : 2024-10-08 20:58:35
- Updated at : 2024-10-12 11:33:47
- Link: https://app-tips.techidaily.com/the-future-of-jobs-with-ai-unveiling-the-endless-possibilities-and-changes-by-zdnet-experts/
- License: This work is licensed under CC BY-NC-SA 4.0.